Images to Maps

Remote sensing images are a grid of pixels with numerical values whereas maps use X and Y coordinates to define the location of points, lines, and areas (polygons) that correspond to map features. Transforming a satellite image into a map that has high accuracy is a process that requires experts and fieldwork.
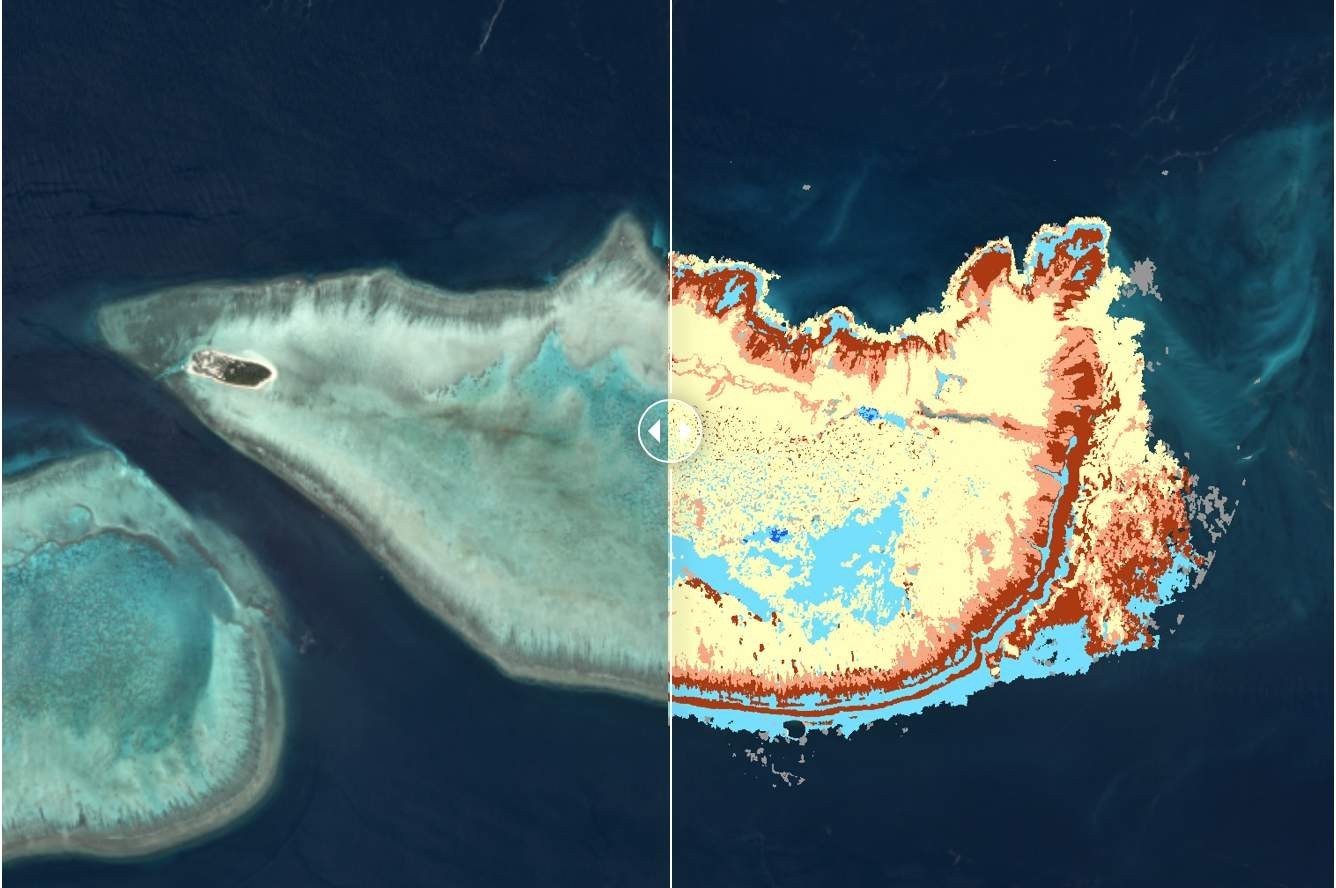
From satellite image (left) to map of the reef with benthic classes (right). This photograph and map depict Australia’s Heron Island, a coral cay at the southern end of the Great Barrier Reef. Image © Allen Coral Atlas
The process to create a map from remote sensing data can be divided into 4 steps that remain similar for all habitats with some differences depending on the type of remote sensing data, the scale, the team doing the mapping, etc.
Some of the most common steps are:
- Acquiring remote sensing imagery
- Processing the raw data
- Training and classification
- Validation
Here we provide a quick overview of each of these steps.
1. Acquiring remote sensing imagery
There are many types of remote sensing data. Optical satellite imagery, radar satellite imagery, hyperspectral imagery from airborne sensors, drone imagery, etc. The remote sensing data used for a project depends on the scale of the project, the capacity for data analysis, the requirements of the habitat, and the budget. For example, mapping a coral reef on a single reef will require different remote sensing data than mapping the global extent of mangroves. As a rule of thumb, the smaller the area to map, the higher the resolution of the images.
2. Processing the raw data
Raw data usually requires processing before it can be used. For example, images to map coral reefs will be corrected for the effects of haze, cloud cover, sun glint, and depth.
3. Training and Classification
Pixel-based supervised is one of the most common image analysis techniques. Pixels representing each class are selected in the mapping scheme that will serve as training pixels. Training pixels have been validated through field data or other reliable sources and a spectral reflectance signature has been created. The training signatures for each class to be mapped are quantitatively compared to each pixel in the image, and pixels are assigned to the mapping class that their spectral signature is most similar to.
Object-based image analysis is a recent and more powerful image classification technique. An object-based image analysis first segments the image into objects. The segmentation is based on the spectral signatures of the pixels, the shape and size of the object, or the texture of the pixels within the object. Once the image is segmented, the user matches each land cover class to a few sample objects. The image is then classified.
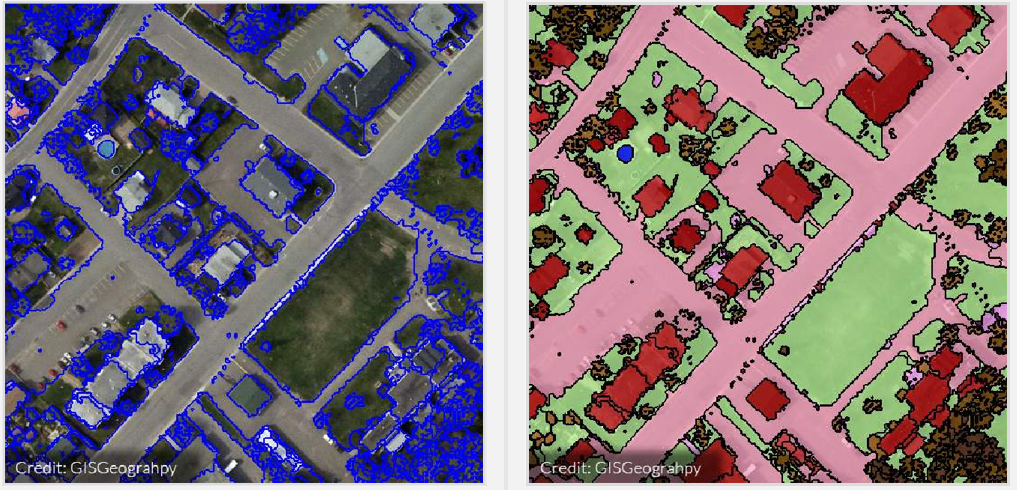
Segmentation and classification of an image during an object-based analysis. Image © GISGeography
4. Validation/Accuracy
An accuracy assessment or validation is an important part of any classification project and provides a measure of how accurate the map product is. It uses independent “ground referenced” data to calculate a statistically based accuracy score based on the comparison of the predicted (mapped) class verses observed class in the field. In other words, the classified pixels or classified objects are compared to reality, or what exists at that location. While independent field data collection can be time consuming and expensive, these data can also be derived from interpreting high-resolution imagery, existing classified imagery, or local experts.